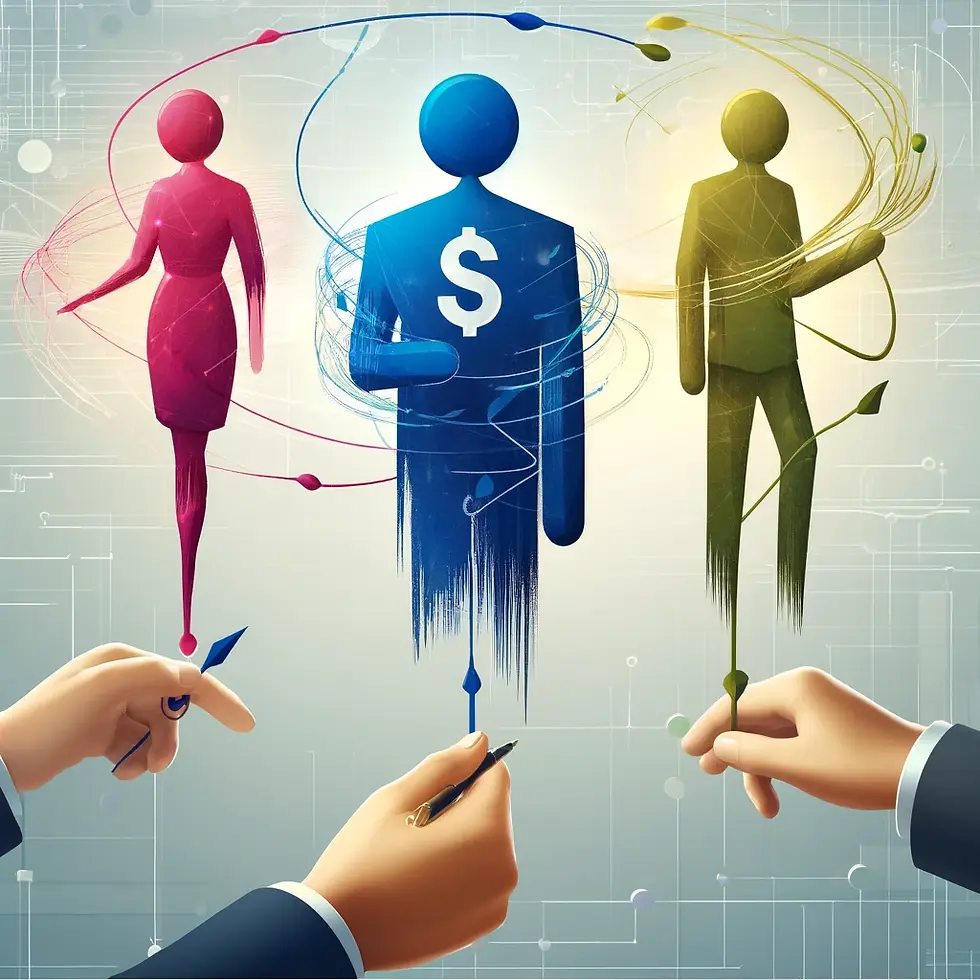
In recent years, market complexity has intensified due to heightened data availability, diverse agent preferences, and rapid shifts in environmental conditions. Complexity Economics, which models aggregated large-group behaviors, often employs Agent-Based Modeling (ABM) to simulate actions and decisions of agents based on specific decision rules. However, traditional ABM approaches sometimes struggle to capture the nuanced, bias-driven nature of individual human decision-making, which falls within the domain of Behavioral Economics—a discipline focused on individual preferences and frequently using Choice Architecture for precise decision guidance.
Definitive Decision Technology, which integrates preference weighting and decision science principles, offers a promising approach to bridge the gap between these disciplines, enhancing ABM with insights from Behavioral Economics. By using pairwise comparisons and the Analytical Hierarchy Process (AHP) pioneered by Thomas Saaty, Definitive can accurately capture utility functions and demand curves to reflect real human preferences and judgments. This process also approximates human “fast” heuristic-based decision-making and properly accounts for cognitive biases.
In this paper, we illustrate how Definitive technology can improve the simulation of complex markets through a previous real estate project in Washington, D.C., where ABM was used to simulate the housing market. We examine how Definitive’s methodology can gather weighted preferences, construct utility functions, and create demand curves for both real and artificial agents within this context. The Washington, D.C. housing market serves as an example application for ABM enhanced by Definitive technology, adding psychological realism by capturing the interaction of buyer, seller, and other agent preferences in a data-driven environment.
About the author: Jeff Hulett leads Personal Finance Reimagined, a decision-making and financial education platform. He teaches personal finance at James Madison University and provides personal finance seminars. Check out his book -- Making Choices, Making Money: Your Guide to Making Confident Financial Decisions.
Jeff is a career banker, data scientist, behavioral economist, and choice architect. Jeff has held banking and consulting leadership roles at Wells Fargo, Citibank, KPMG, and IBM.
Background: Agent-Based Modeling in Real Estate
Agent-based modeling simulates the behaviors and interactions of individual agents within a specific environment, making it suitable for studying complex, dynamic systems like housing markets. In a housing ABM, agents can represent various stakeholders—homebuyers, sellers, mortgage lenders, real estate agents, and developers. Each agent follows a set of rules based on their individual goals, preferences, and available information, allowing the model to reproduce complex phenomena such as housing price bubbles, shifts in market demand, and impacts of regulatory policies.
A recent ABM study of the D.C. housing market by Axtell, Farmer, and others modeled agents using a mix of statistical data and economic decision rules. This approach successfully replicated certain market trends but also highlighted areas for improvement, particularly around capturing the “fuzzy,” human side of decision-making, where preferences, biases, and emotional factors play significant roles. This model omitted certain real-world factors that can significantly influence agent decisions, such as seasonal market variations and the role of real estate agents and mortgage brokers. These professionals often shape buying behavior and loan accessibility, impacting both the flow of information and the range of options available to agents. Here, Definitive Decision Technology can play a transformative role by integrating these perspectives into the ABM, allowing the model to more accurately reflect market dynamics.
The Definitive Approach: An Overview
Definitive Decision Technology specializes in gathering weighted preferences to model decision-making in a highly realistic way. The approach relies on the AHP, a decision-making tool developed by Thomas Saaty, which uses pairwise comparisons to rank criteria based on importance. By asking decision-makers to compare criteria two at a time, AHP captures the relative weight of each criterion, creating a robust preference structure. These weighted preferences can reveal, for instance, that a buyer might prioritize proximity to a good school over having a large yard, or vice versa.
Additionally, Definitive uses a process to approximate “fast” heuristic-based decision-making, which is particularly relevant in markets where agents often rely on intuition or “gut feelings.” By structuring decisions in a way that properly accounts for cognitive biases—such as overconfidence or loss aversion—Definitive’s technology can approximate the strengths and limitations of human judgment. The final outcome is a utility function that more closely mirrors real-world preferences, enabling the creation of demand curves suitable for ABM.
The Definitive approach further addresses the limitations seen in previous ABM studies by enabling the inclusion of perspectives from key stakeholders, such as real estate agents and mortgage brokers. These stakeholders directly influence the buying process by providing information, negotiating terms, and facilitating loan approval processes. By integrating their perspectives, Definitive’s process enhances the accuracy of ABM, capturing both direct buyer preferences and the indirect effects of market facilitators.
Applying Definitive Technology to an ABM Model of the D.C. Real Estate Market
To illustrate how Definitive technology could enhance ABM for an enhanced D.C. real estate project, let us consider a simulation model where potential homebuyers (agents) are deciding between various housing options in different neighborhoods. Each agent has a set of preferences (criteria) that they use to evaluate housing options. These preferences include location, price, proximity to work, school quality, neighborhood safety, and property size.
Step 1: Defining and Weighting Preferences Using Definitive Decision Technology, we start by defining and weighting agent preferences through pairwise comparisons. This approach asks agents to compare preferences two at a time, capturing their relative importance and establishing a personalized weighting structure. The serial pairwise trade-off approach is important. This best replicates our heuristic-based judgment in a way that optimizes the accuracy of the judgment in the context of overall utility. For homebuyers in the D.C. market, preferences might include factors like proximity to public transportation, school quality, and property size. The resulting weights are then applied to construct individual utility functions, which drive agents’ decision-making processes. This is where the Analytical Hierarchy Process is implemented using the matrix algebra -- eigenvectors and eigenvalues -- mathematical process invented by Thomas Saaty.
Step 2: Building Utility Functions With weighted preferences established, the next step is to construct utility functions that represent each agent’s decision-making criteria. Utility functions are mathematical representations of an agent’s preferences, translating complex decisions into quantifiable metrics. In the housing market example, these utility functions allow agents to rank housing options based on the degree to which they meet weighted criteria, such as affordability and commute distance. By capturing preferences in this structured way, the ABM can simulate how agents select options that maximize their utility. For example, a utility function for an agent might take the form:
U = w1 × Location + w2 × Price + w3 × School Quality + w4 × Safety + w5 × Property Size
where w1,w2,…,w5 are the weights assigned to each criterion based on the pairwise comparisons. Weights will add to 1.
See the appendix for clarification of utility maximization and rationality.
Step 3: Constructing Demand Curves Once utility functions are in place, we aggregate them to create demand curves. Each agent’s individual utility function reflects their willingness to pay for specific property features. Aggregating these functions provides an overall demand profile for the market. For example, in D.C., high demand for homes near metro stations could be identified as a trend, reflecting both preference weights and utility calculations from all agents in the model. This demand curve is dynamic and can adjust based on interactions and changing conditions, simulating real market behavior. It is expected that demand curves will differ by cultural heritage, gender, income, and other social dimensions. Because this approach is bottoms up, with the "atomic" level being the individual agent, the demand curves can be aggregated by almost any dimension.
Step 4: Incorporating Real Estate Agents and Mortgage Brokers Incorporating real estate agents and mortgage brokers into the model addresses an important limitation found in previous ABM studies. These professionals play crucial roles in shaping housing market dynamics by guiding buyers, negotiating terms, and facilitating access to financing. With Definitive’s process, the perspectives and preferences of these stakeholders can be integrated into the ABM. For instance, real estate agents could act as intermediary agents, filtering and recommending properties based on buyers’ preferences, while mortgage brokers might adjust loan availability and interest rates depending on the market’s risk profile. This inclusion adds realism to the model and provides insight into how these roles influence market outcomes.
Step 5: Accounting for Cognitive Biases Cognitive biases significantly impact decision-making, especially in complex markets. Definitive technology applies a preference-weighting process that adjusts for common biases, such as availability bias, where recent events disproportionately affect judgments, or overconfidence, where agents overestimate the accuracy of their decisions. By accounting for these biases, Definitive’s approach makes the ABM more realistic, capturing the imperfections in human decision-making. For instance, a buyer may overvalue certain properties due to emotional attachment, while sellers may be influenced by anchoring on recent listing prices.
Step 6: Generating Artificial Agents Definitive’s methodology allows for the creation of artificial agents with realistic preferences based on aggregated data. These agents can serve as representative samples of broader population segments, enabling the ABM to simulate behaviors across demographic or geographic groups. In the D.C. housing project, artificial agents could represent specific types of buyers (e.g., first-time buyers, investors) and follow decision rules calibrated from historical data. This approach enhances the model’s scalability, allowing it to approximate the actions of large populations based on weighted preferences and utility functions.
Step 7: Enabling Dynamic Interactions Between Agents A critical strength of ABM is its ability to simulate interactions between agents and dynamic adjustments in demand and supply sentiment. Definitive’s approach allows buyers and sellers to interact over time, adjusting their preferences and price expectations based on market feedback. For instance, a seller may list their home above market value but lower it over time if they receive no offers, while a buyer may adjust their price threshold based on inventory levels and available financing options. By simulating these interactions, the ABM captures the evolving dynamics of supply and demand, providing a nuanced view of market behavior that includes adjustments in real-time sentiment. This feature is especially valuable in fluctuating markets, where agent interactions drive trends and help identify emerging patterns.
Generalizing the Definitive Approach for Agent-Based Modeling
Definitive offers an app called Definitive Choice, currently available on Apple (iPhone) and Google (Android) platforms. The app has been in production for five years and includes over 50 utility model templates, such as the homebuying template, allowing it to support a broad range of decision-making simulations. Integrated with GenAI, Definitive Choice accelerates agent users' interactions without compromising quality. As an ABM simulation technology, it enables the distribution across large agent groups with ease, while an administrative backend provides detailed reports and access to comprehensive interaction data from agent users.
These seven steps define a broad, adaptable framework for applying Definitive technology across ABM projects, beyond just the D.C. housing market example. By beginning with the identification and weighting of preferences, the Definitive approach builds utility functions and demand curves that mirror real-world behavior. It captures both the structured, data-driven aspects of decision-making and the psychological components like heuristic judgments and cognitive biases. Through this combination, ABM becomes well-suited to reflect the complexities of human decision-making across diverse applications. Artificial agents simulate general patterns, enabling the methodology to be applied across fields such as urban planning, public health, and finance, where agent interactions and preference dynamics are critical for realistic simulations.
Conclusion
Definitive Decision Technology’s structured approach to capturing and weighting preferences, constructing utility functions, and creating demand curves is well-suited for enhancing agent-based modeling. By integrating the perspectives of influential market participants, such as real estate agents and mortgage brokers, Definitive technology addresses key limitations of previous models, adding layers of realism to ABM in the D.C. housing market. This comprehensive, seven-step methodology enhances ABM projects by simulating both individual agent behavior and dynamic interactions between agents, providing accurate, real-time market insights.
The framework’s generalizable design further broadens its applicability across various fields, making it a versatile solution for complex agent-based simulations. As shown in the D.C. housing market case, the Definitive approach can deliver more realistic, data-driven insights into agent decision-making, with the potential to transform how ABM models are constructed and applied across industries.
Appendix: Clarifying Utility Maximization in the Context of Diverse Rationality
The concept of “maximizing utility” in Step 2 is not intended to imply the traditional economic assumption of a single, convergent rational outcome for all agents. Instead, this framework embraces the principle of diverse rationality, which acknowledges that rationality is personal, context-dependent, and shaped by bounded rationality—agents make decisions within the constraints of limited information, cognitive biases, and situational factors.
For instance, a homebuyer may prioritize location over price in one scenario but shift focus to affordability due to financial constraints or changing family dynamics. These dynamic preferences reflect the real-world diversity in decision-making, and utility functions in the ABM approximate each agent’s unique rationality at a given moment.
Choice architecture tools like Definitive Choice play a critical role in capturing this diverse rationality across larger groups, enabling the modeling of preferences that reflect the complexity and adaptability of real-world decision-making at scale.
See: Hulett, J. (2024). Becoming Behavioral Economics: The Social Science Revolutionizing Decision-Making. The Curiosity Vine.
Resources for the Curious
i. Saaty, T. L. (1980). The Analytic Hierarchy Process: Planning, Priority Setting, Resource Allocation. New York: McGraw-Hill.
ii. Tesfatsion, L., & Judd, K. L. (Eds.). (2006). Handbook of Computational Economics, Volume 2: Agent-Based Computational Economics. Amsterdam: Elsevier.
iii. Axtell, R. L., & Farmer, J. D. (2023). "Agent-Based Modeling in Economics and Finance: Past, Present, and Future." Journal of Economic Literature.
iv. Geanakoplos, J., Axtell, R., Farmer, J. D., Howitt, P., Conlee, B., Goldstein, J., Hendrey, M., Palmer, N., & Yang, C.-Y. (2012). "Getting at Systemic Risk via an Agent-Based Model of the Housing Market." American Economic Review, 102(3), 53–58.
v. Axtell, R., Farmer, J. D., Geanakoplos, J., Howitt, P., Carrella, E., Conlee, B., Goldstein, J., Hendrey, M., Kalikman, P., Masad, D., Palmer, N., & Yang, C.-Y. (2014). "An Agent-Based Model of the Housing Market Bubble in Metropolitan Washington, D.C." SSRN Electronic Journal.
vi. Miller, J. H., & Page, S. E. (2007). Complex Adaptive Systems: An Introduction to Computational Models of Social Life. Princeton: Princeton University Press.
vii. Iacoviello, M. (2005). "House Prices, Borrowing Constraints, and Monetary Policy in the Business Cycle." American Economic Review, 95(3), 739-764.
viii. Saaty, T. L. (1980). The Analytic Hierarchy Process: Planning, Priority Setting, Resource Allocation. McGraw-Hill.
ix. Hulett, J. (2023). "The Subtleties of Lending Discrimination." The Curiosity Vine.
x. Farmer, J. D., & Foley, D. (2009). "The Economy Needs Agent-Based Modeling." Nature, 460(7256), 685-686.
xi. Epstein, J. M., & Axtell, R. (1996). Growing Artificial Societies: Social Science from the Bottom Up. MIT Press.
xii. Hulett, J. (2023). "Affordable Housing Solutions." The Curiosity Vine.
xiii. Lux, T. (2009). "Agent-Based Models of Financial Markets." In Hens, T. & Schenk-Hoppé, K. R. (Eds.), Handbook of Financial Markets: Dynamics and Evolution. Elsevier.
Comentarios