"Listen to your customer!" and other bank behavioral testing takeaways
- Jeff Hulett
- Mar 30, 2021
- 3 min read
Updated: Jan 24, 2022
Using behavioral economics approaches to optimize consumer banking operations.
By Jeff Hulett, March 27, 2021
The following is an excerpt from our article Every bank needs a nudge....
Earlier in my career, I was a behavioral economist in large banking and consumer lending organizations. I led BIT - like / nudge unit teams. (1) Our job was to use a wide range of analytical techniques (some AI related), data sources, Randomized Control Trial (RCT) techniques, and other behavioral techniques to manage bank credit loss exposure and optimize lending program performance. We were large enough that we had our own testing operation, which meant we had dedicated customer agents trained for behavioral testing. We also had systems designed to integrate results with the test design parameters. These were some of the coolest jobs I ever had!
Based on my nudge unit experiences, the following are some important takeaways:
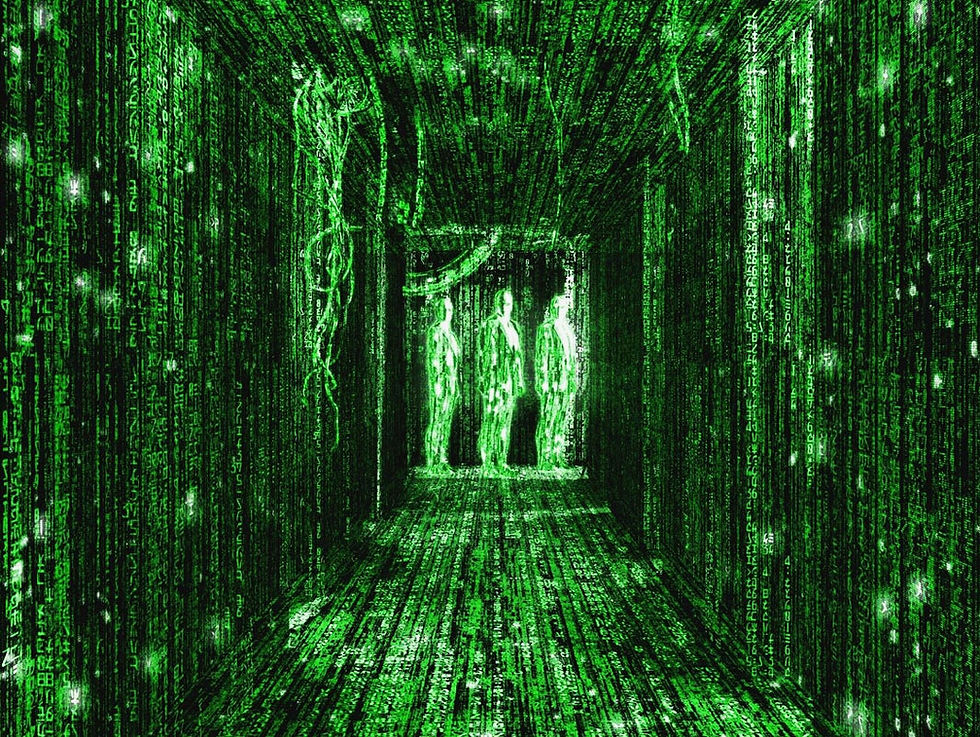
Listen to your customers! Go beyond ”The Matrix" data view of your customers. Have customer round tables and focus groups. Great testing ideas come from listening to your customers. Personally, I think all data scientists and related should have some kind of regular customer interaction.
Seek unique data about your customers. This could be an insight from existing data or it could be new and unique data.
Some "data digging" is ok. Data Scientists often do not like data digging. Data digging is code for the messy ETL related data processes needed for less structured data sets. It can be grinding work. I call this the "meta meta data." That is, the story behind the data dictionary. It can be time consuming and take away from primary data analysis. While I hope Data Scientists spend the majority of their time analyzing, some data digging can be both instructive and can lead to a "digging for gold" outcome by finding unique competitive insights.
Test new Artificial Intelligence techniques. My observation is, usually new analytical techniques are not always better than "tried and true" techniques like Regression and Decision Trees. However, we always learn something new and useful in the process, beyond the fact that new AI techniques were not always effective. It is worth the exploration, just not for the reasons you may expect.
Test execution is critical. Commit the resources to proper test execution. Testing systems may include 1) testing program guides, 2) coding to differentiate test and control groups, 3) collection of performance results, 4) agent or customer scripting, 5) availability of characteristic data and related testing information. 6) Analytical resources to analyze and provide post test results and recommendations.
Test with a success scaling outcome in mind. Meaning, assuming success, how will this test be rolled out and scaled in our base business? Unfortunately, I know successful tests that failed to impact business results. This occurred because of a failure to scale.
Causality is key! RCT is necessary to drive confidence in the causal nature of your results. It will also help business leaders understand the value of risk testing. Often, a small percentage risk test gain will lead to a significant bottom line improvement. By the way, not every test is suitable for RCT. If you test without a control, be very explicit about what you hope to learn and the likely learning limitations.
Useful for all financial services products. While this example is likely most useful in credit card context, with today's information technology, it can easily be useful for all financial services products.
Note
(1) "BIT" stands for Behavioural Insights Team. Please see our article for more specifics - Every bank needs a nudge...
Comments