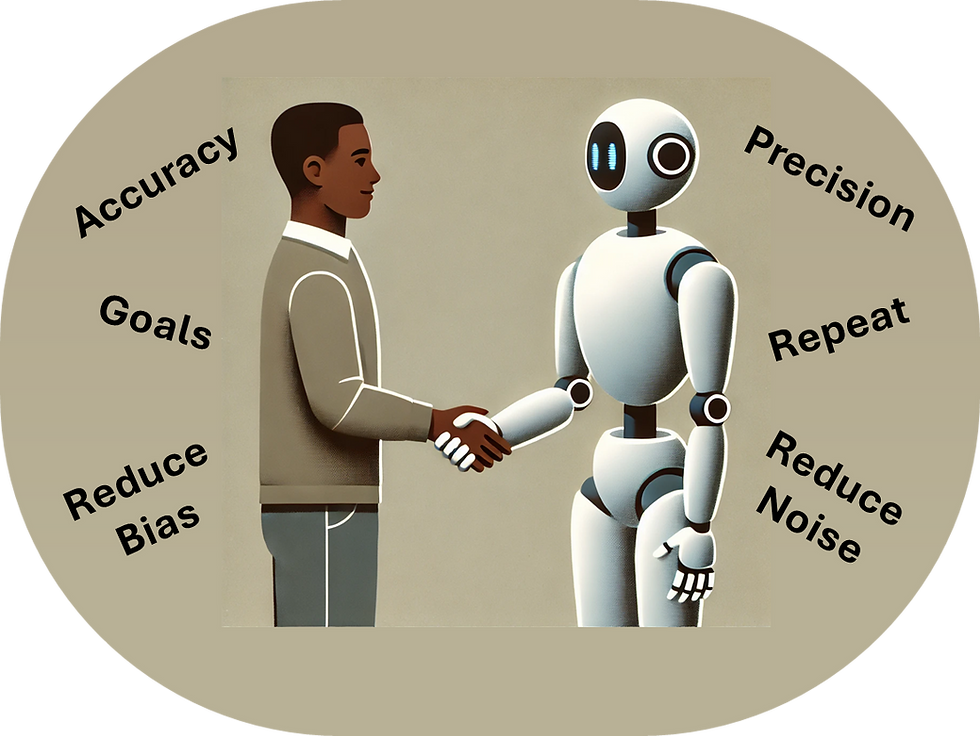
In today’s AI-driven world, partnering effectively with Generative AI (GenAI) can greatly amplify results. However, understanding the difference between accuracy and precision is essential to maximize the potential of this partnership. Accuracy aligns GenAI with your specific goals, while precision ensures consistency. When combined, they empower you to achieve impactful, reliable results. I conclude with six practical suggestions for getting the most out of your partnership with GenAI, along with recommended resources for those who wish to explore this topic further.
About the author: Jeff Hulett leads Personal Finance Reimagined, a decision-making and financial education platform. He teaches personal finance at James Madison University and provides personal finance seminars. Check out his book -- Making Choices, Making Money: Your Guide to Making Confident Financial Decisions.
Jeff is a career banker, data scientist, behavioral economist, and choice architect. Jeff has held banking and consulting leadership roles at Wells Fargo, Citibank, KPMG, and IBM.
Accuracy: Reducing Bias for Clear, Goal-Oriented Results
Accuracy in AI means achieving the intended target. It involves reducing bias by setting clear goals and aligning processes with those goals. Think of accuracy as the compass guiding GenAI toward relevant results. Without accuracy, output may appear refined but lack relevance. GenAI’s role is to produce the requested content, but it is the human partner’s responsibility to define the goal, ensuring results are on target and useful.
Despite GenAI’s impressive capabilities, it lacks the context to independently understand goal nuances. For example, if you ask GenAI for information without a clear goal, it may generate responses that are detailed yet misaligned with your intent. Providing context ensures GenAI delivers accurate, valuable results. By clarifying your objectives, you minimize the risk of irrelevant content.
Precision: Reducing Noise through Consistency in Results
Precision, meanwhile, is about consistency. It is the ability to generate repeatable, noise-free results across similar queries. Think of precision as a tool for achieving the same result each time, ensuring consistent quality. When applied to GenAI, precision minimizes variations, providing stability in outputs.
GenAI is highly precise, delivering predictable results for repeated prompts. This precision is ideal for tasks requiring structured responses, summaries, or data extraction. However, precision alone does not guarantee relevance; without accuracy, even a consistently executed process might miss the mark. Precision strengthens the reliability of GenAI’s output but must be guided by context for truly useful results.
The Human-AI Partnership: Combining Accuracy with Precision for Maximum Impact
A successful GenAI partnership combines AI’s precision with human-defined accuracy. Humans set the goals, add context, and reduce bias, ensuring that each response aligns with the intended purpose. GenAI, in turn, brings powerful precision, generating consistent responses that enhance efficiency and productivity.
While GenAI often presents results with a high degree of confidence, suggesting precision, it may not always be correct. Human oversight is essential to verify these results and avoid potential errors. For example, if a researcher uses GenAI to gather insights, the tool may produce responses that seem precise. However, the researcher must review the output carefully to ensure its reliability and relevance. Together, they create a result that is goal-aligned, repeatable, and free from irrelevant noise.
Conclusion
In an effective GenAI partnership, understanding accuracy and precision unlocks AI’s true value. GenAI offers reliable, noise-free responses, while the human partner ensures every output is anchored to the desired goal. By merging human context with AI’s precision, GenAI becomes a powerful tool for achieving accurate, bias-free, and consistent results. This partnership amplifies efficiency, transforming AI into an invaluable asset for informed decision-making and actionable outcomes.
Appendix: Top 6 Suggestions for Getting the Most Out of Your GenAI Partnership
Define Clear Goals from the Start Be specific about your objectives. GenAI performs best when given a well-defined task. Clear goals allow it to align its precision with your intended outcome.
Provide Context with Your Queries GenAI lacks an inherent understanding of context, so ensure your prompts are detailed and relevant to the topic. Adding context can help it generate responses that are more tailored and useful.
Review Outputs for Precision GenAI’s responses may appear confident and precise, but they’re not always correct. Always review its output to confirm that it aligns with your original goals and is free from errors or inconsistencies.
Curate Credible Information for GenAI Enhance GenAI’s output by supplying curated information from reliable sources. By sharing data or context from reputable references, you improve the quality and relevance of GenAI's responses, helping it to produce results that align more closely with established facts and trusted insights.
Use Iterative Prompting Refine your prompts if the initial responses don’t meet expectations. Iterative prompting helps steer GenAI closer to what you need, improving both accuracy and relevance over time.
Leverage GenAI’s Strengths in Consistency GenAI excels at generating repeatable, structured responses. Use it for tasks that benefit from this precision, such as summarizing information, generating lists, or analyzing repetitive data.
By following these suggestions, you can maximize the effectiveness of your partnership with GenAI, achieving both accuracy and precision for better, more actionable results.
Resources for the Curious
Next are some of my favorite resources on the topic.
Kahneman, D., Sibony, O., & Sunstein, C. R. (2021). Noise: A Flaw in Human Judgment. This book examines the concept of "noise" as unwanted variability in human judgments and decision-making processes, contrasting it with bias, which is a systematic deviation. Kahneman and colleagues provide a framework that helps readers understand how reducing both noise and bias can lead to more accurate and precise outcomes.
S. Zhang, P.R. Heck, M.N. Meyer, C.F. Chabris, D.G. Goldstein, J.M. Hofman (2023) "An illusion of predictability in scientific results: Even experts confuse inferential uncertainty and outcome variability." Proc. Natl. Acad. Sci. U.S.A. 120 (33) e2302491120, https://doi.org/10.1073/pnas.2302491120 (2023). This study explores how experts can misinterpret inferential uncertainty as outcome variability, highlighting the need to clearly distinguish between precision (noise reduction) and accuracy (bias reduction) in scientific research and AI applications.
Tversky, A., & Kahneman, D. (1974). "Judgment Under Uncertainty: Heuristics and Biases." Science, 185(4157), pp. 1124-1131. This classic article is foundational in the study of biases in human decision-making. While it primarily focuses on bias, it provides essential context for understanding why accuracy (freedom from bias) is critical in any decision-making framework, including AI applications.
Geman, S., Bienenstock, E., & Doursat, R. (1992). "Neural Networks and the Bias/Variance Dilemma." Neural Computation, 4(1), pp.1-58. This paper introduces the bias-variance trade-off in machine learning, a key concept that explores the balance between accuracy (bias) and variability (noise or variance) in model predictions. It is a fundamental resource for understanding how to adjust AI algorithms for better accuracy and precision.
Gigerenzer, G. (2008). Rationality for Mortals: How People Cope with Uncertainty. Gigerenzer's work focuses on the practical aspects of decision-making under uncertainty and variability (noise) in human judgment. The book highlights strategies for improving judgment and decision-making by addressing noise, making it a practical complement to AI research on precision.
Comments